Keynote talk via Livestream: Machine Learning in Exoplanet Characterisation with Dr Ingo Waldmann Event
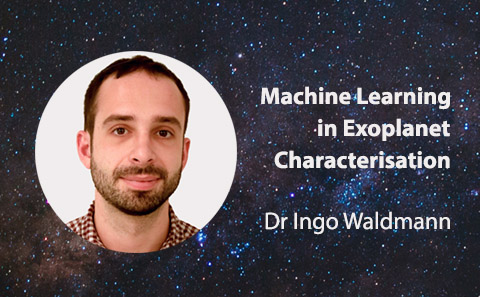
- Time:
- 10:15 - 11:00
- Date:
- 30 June 2023
- Venue:
- Online via livestream
Event details
As part of a larger invitation only event hosted by the Universities of Southampton and Strathclyde, we are inviting you to watch a keynote talk via livestream with Associate Professor in Astrophysics at University College London, and Turing Fellow, Dr Ingo Waldmann.
Abstract
The exploration of extrasolar planets, which are planets orbiting stars other than our own, holds great potential for unravelling long-standing mysteries surrounding planet formation, habitability, and the emergence of life in our galaxy. By studying the atmospheres of these exoplanets, we gain valuable insights into their climates, chemical compositions, formation processes, and past evolutionary paths. The recent launch of the James Webb Space Telescope (JWST) marks the beginning of a new era of high-quality observations that have already challenged our existing understanding of planetary atmospheres. Over its lifetime, the JWST will observe approximately 50 to 100 planets. Furthermore, in the coming decade, the European Space Agency's Ariel mission will build on this progress by studying in detail the atmospheres of an additional 1000 exoplanets.
In this talk, I will outline three fundamental challenges to exoplanet characterisation that lend themselves well to machine-learning approaches. Firstly, we encounter the issue of extracting useful information from data with low signal-to-noise ratios. When the noise from instruments surpasses the signal from exoplanets, we must rely on self-supervised deconvolution techniques to learn accurate instrument models that go beyond our traditional calibration methods. Secondly, in order to interpret these alien worlds, we must employ highly complex models encompassing climate, chemistry, stellar processes, and radiative transfer. However, these models demand significant computational resources, necessitating the use of machine learning surrogate modelling techniques to enhance efficiency. Lastly, the Bayesian inverse problem, which traditionally relies on methods like Markov Chain Monte Carlo (MCMC) and nested sampling, becomes particularly challenging in high-dimensional parameter spaces. In this regard, simulation-based inference techniques offer potential solutions.
It is evident that many of the modelling and data analysis challenges we face in the study of exoplanets are not unique to this field but are actively investigated within the machine learning community. However, interdisciplinary collaboration has often been hindered by jargon and a lack of familiarity with each other's domains. In order to bridge this gap, as part of the ESA Ariel Space mission, we have successfully organized four machine learning challenges hosted at ECML-PKDD and NeurIPS (https://www.ariel-datachallenge.space). These challenges aim to provide novel solutions to long-standing problems and foster closer collaboration between the exoplanet and machine learning communities. I will end this talk with a brief discussion of the lessons learned from running these interdisciplinary data challenges.
About the speaker
Dr Ingo Waldmann completed his PhD at the University College London (UCL) in 2012 and is now an Associate Professor in Astrophysics at UCL. His work focuses on the development of machine learning and inverse modelling in exoplanet -planets around other stars- and solar system sciences.
He is the PI of the European Research Council and UK Space Agency funded ExoAI group, the AI lead of the European Space Agency (ESA) Ariel Space Mission and the ESA_lab AI representative at UCL. He is the Deputy Director of the UCL Centre for Space Exochemistry Data (CSED) focusing on AI applications across space industries and the Director of the UCL Planetary Sciences MSc programme.
He was awarded the Royal Astronomical Society Fowler Award in 2019 for his work on machine learning in exoplanetary science and elected a Turing Fellow in 2021. He is a co-lead of the Space Science Interest Group at The Alan Turing Institute. In 2020, he co-founded Spaceflux Limited, a startup building a global robotic telescope network to track the impact of man-made satellites and space debris.
This event is supported by The Alan Turing Institute. The Turing is not involved in the agenda or content planning.