S3RI Seminar - Joint mean-covariance modelling and its R package: jmcm, by Jianxin Pan (Manchester) Seminar
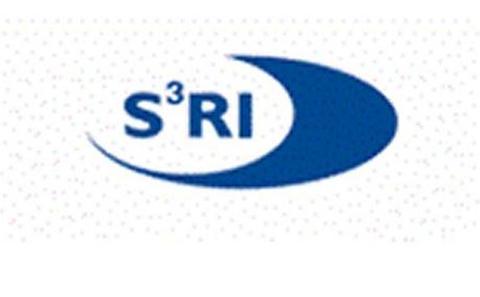
- Time:
- 14:00 - 15:00
- Date:
- 11 May 2017
- Venue:
- Room 5027, Lecture Theatre 5A, Building 54, Mathematical Sciences, University of Southampton, SO17 1BJ
For more information regarding this seminar, please telephone Professor Dankmar Boehning on 023 8059 6712 or email D.A.Bohning@southampton.ac.uk .
Event details
Longitudinal studies commonly arise in various fields such as psychology, social science, economics and medical research, etc. It is of great importance to understand the dynamics in the mean function, covariance and/or correlation matrices of repeated measurements. However, high-dimensionality (HD) and positive-definiteness (PD) constraints are two major stumbling blocks in modelling of covariance and/or correlation matrices. It is evident that Cholesky-type decomposition based methods are effective in dealing with HD and PD problems, but those methods were not implemented in statistical software yet, making a difficulty for practitioners to use. In this talk, three Cholesky decomposition based methods for joint modelling of mean and covariance structures, namely Modified Cholesky decomposition (MCD), Alternative Cholesky decomposition (ACD) and Hyperpherical parameterization of Cholesky factor (HPC), will be introduced. The newly developed R package jmcm containing the MCD, ACD and HPC methods will then be introduced. Demonstration will be made by running the package jmcm and comparison of those methods will be made through analyzing real data examples. This is joint work with Yi Pan (The University of Manchester)
Speaker information
Professor Jianxin Pan, School of Mathematics, University of Manchester. Research and projects include Joint modelling of longitudinal and survival data analysis; Modelling of mean-covariance structures; Modelling of high-dimensional and complex data; Variable selection in various statistical models; Parametric and nonparametric models for clustered data; Functional data models; Statistical diagnostics.