Research projects
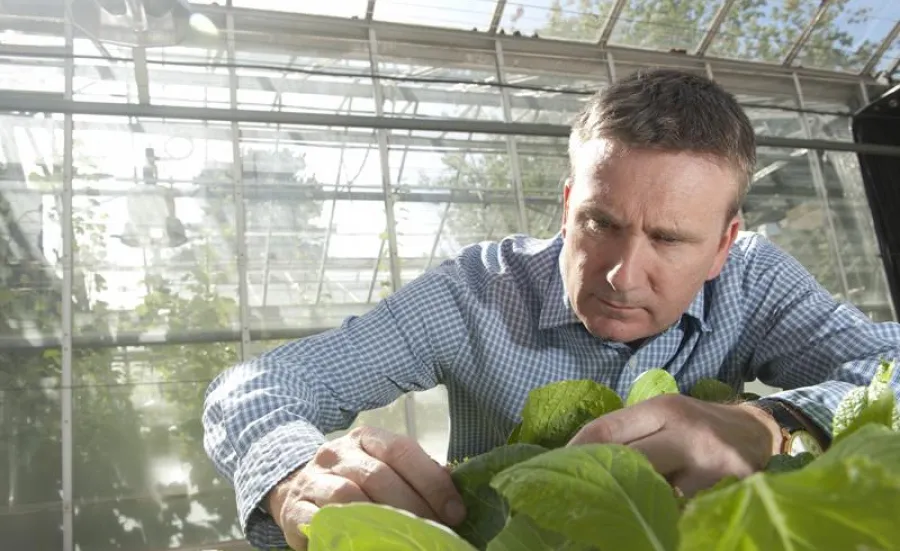
By collaborating across different disciplines and subjects, our university researchers are taking on new projects to solve challenges in the modern world.
By collaborating across different disciplines and subjects, our university researchers are taking on new projects to solve challenges in the modern world.